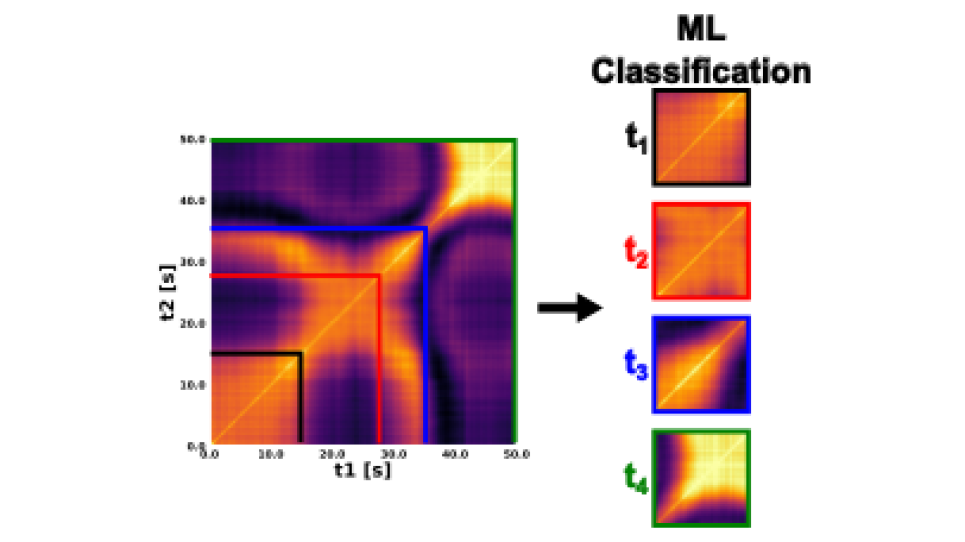
Figure 1. The AI-NERD framework is used for unsupervised analysis of high data rate x-ray science experiments. Unsupervised classification enables automated tracking of microscopic dynamics and moves towards real-time detection of rare events.
AI-informed analysis of X-ray science data extends experimental capabilities, guides scientific discovery, and enables precise analysis of materials dynamics in the big data regime.
Improvements in detector hardware, and increased x-ray flux and coherence after the APS-U will enable high-performance in-situ studies of functional materials to elucidate how microscopic changes in a materials structure can influence macroscopic properties and performance. However, these experiments will generate several gigabytes of data per second, and terabytes of data per experiment. To further complicate matters, dynamics in out-of-equilibrium system produce data which appear chaotic and nearly impossible to understand. Automated classification of X-ray science data represents a first step towards addressing both the data production bottleneck, and the development of quantitative methods for describing dynamics in complex materials.
Our Artificial Intelligence for Non-Equilibrium Relaxation Dynamics (AI-NERD) framework is a deep learning model trained on the LCRC’s Swing GPU cluster to aid the real-time analysis of experimental X-ray science data. AI-NERD is used to distill large experimental datasets into a feature-rich subspace where unsupervised clustering and visualization algorithms are used to uncover the time-dependent behavior of the material. This flexible workflow accelerates the exploration of X-ray science data without requiring any underlying assumptions about the data, enabling scientific discovery in a material- and process-agnostic manner.
Reference:
Horwath, J.P., Lin, X.M., He, H., Zhang, Q., Dufresne, E.M., Chu, M., Sankaranarayanan, S.K., Chen, W., Narayanan, S. and Chreukara, M.J., 2022. Elucidation of Relaxation Dynamics Beyond Equilibrium Through AI-informed X-ray Photon Correlation Spectroscopy. arXiv preprint arXiv:2212.03984.